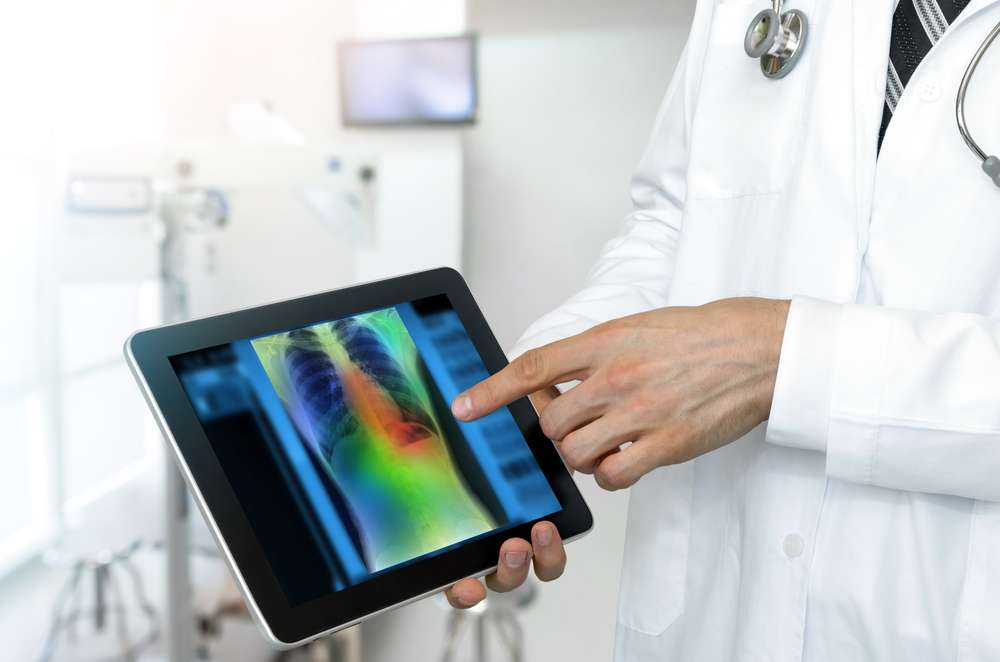
The Current applications and future perspectives of Artificial Intelligence (AI) in lung cancer
In recent years, AI research, particularly in thoracic imaging, has evolved. AI aids in virtual biopsies and treatment response prediction. Numerous detection, characterization, and stratification tools have been introduced, with some now available commercially.
The integration of AI tools into clinical practice marks the progression of medical imaging digitization. Recent years have witnessed a surge in AI adoption, particularly with the advent of deep learning. While initial concerns during the AI hype speculated about the replacement of radiologists, we have now transitioned to an adoption phase. Among medical specialties, thoracic imaging has been a significant beneficiary of AI and deep learning advancements. AI has been instrumental in quantifying, characterizing, and monitoring interstitial lung disease, and bronchial conditions, and addressing the challenges posed by COVID-19.
Furthermore, AI enables virtual biopsies for fragile patients, especially before stereotactic radiotherapy or selecting optimal surgical timing for subsolid nodules, which can be pre-invasive or invasive lesions. Radiomic and deep-learning methods aid in differentiation. AI is also used to predict genetic mutations (e.g., EGFR) and immunotherapy responses, enhancing personalized treatments. Mortality risk stratification, using deep learning, predicts 2-year overall survival based on chest CT scans. While AI offers valuable insights for lung cancer, these tools are not yet a substitute for biopsy or primary treatment decisions.
In the dynamic field of lung cancer AI, various detection, characterization, and stratification tools have been proposed, with some commercially available. For future lung cancer screening programs, AI-based tools may mitigate medico-economic challenges and improve screening efficiency by minimizing false negatives and false positives.
Reference Link: