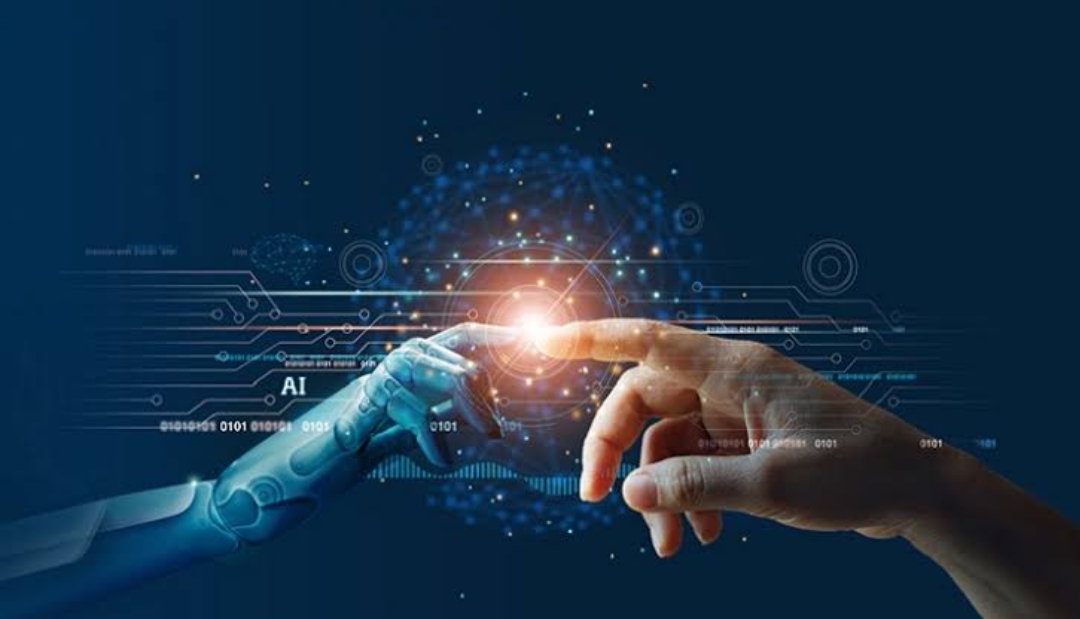
Deep Learning–Based Algorithm for Detecting Aortic Stenosis
A machine learning algorithm detected severe aortic stenosis using audio files from a set of patients with accuracy similar to board-certified cardiologists, according to findings from a proof-of-concept study.
“Artificial intelligence will be the upcoming technology to assist doctors in standard techniques like auscultation in the clinical routine,” Heinrich Wieneke, MD, professor and head of the department of cardiology at Elisabeth-Krankenhaus Essen, Germany. “The present study gives proof of the concept that AI‐assisted auscultation can provide results on a high-expert level concerning the detection of aortic stenosis.”
Wieneke and colleagues trained a deep neural network with preprocessed audio files of 100 patients with significant aortic stenosis and 100 controls without aortic stenosis, and then evaluated its performance with a test dataset of 40 patients. The primary outcome measures were sensitivity, specificity and F1-score. Researchers compared the results of the deep neural network with the performance of 10 cardiologists, 10 residents and 10 medical students.
The findings were published in Clinical Cardiology.
Researchers found that 18% of patients without aortic stenosis and 22% of patients with aortic stenosis showed an additional moderate or severe mitral regurgitation. The deep neural network showed a sensitivity of 0.9 (95% CI, 0.81-0.99), a specificity of 1 and an F1‐score of 0.95 (95% CI, 0.89-1) for the detection of aortic stenosis.
Researchers calculated an F1‐score of 0.94 for cardiologists (95% CI, 0.86-1), 0.88 for residents (95% CI, 0.78-0.98) and 0.88 for students (95% CI, 0.78-0.98).
“Integrating this technology into an electronic stethoscope could be the next step to upgrade this system for everyday clinical use,” Wieneke. “A stethoscope that indicates a warning in the event of certain valve defects would be conceivable in the foreseeable future.”
The researchers noted that few patients with moderate aortic stenosis were included, as the study was conducted with data from patients admitted to a tertiary teaching hospital for specialized valve therapy. Patients with other valvular diseases like hypertrophic obstructive cardiomyopathy were also not included, and few patients with pure mitral regurgitation participated.
Thus, the presented algorithm is far from perfect, and this study can only be the first step in introducing artificial intelligence for valvular heart disease into everyday clinical practice,” the researchers wrote. On the other side, it also shows that artificial intelligence can, in principle, be helpful in the auscultation of heart sounds.
Visit DocMode for Courses and lectures